Today most of the companies depend on data. It is the lifeblood of most of the enterprises. Insights derived from organizational data drives major and minor decisions, provides incremental boosts in overall performance and drastic boosts occasionally. However; if all this is like rocket science and your company does not see such results from the data, it’s not a problem with analytics. It means that your organizational data management teams are missing out on some important key steps in preparing the data for analytics. Enlisted are a few more scenarios; if your company encounters any of these, you are in for a real problem.
- How do you behave when it comes to decision-making?
- Do you spend a long time thinking over every single decision?
- Are you sometimes afraid of making the wrong decision?
- Do you feel a need to analyze every single option before you come to a conclusion?
- Does your over-analysis often stop you from making a move quickly — at times missing perfectly good opportunities?
Only with quality data preparation, an enterprise can gain the ability to access both internal and external data sources, and transform them into conveniently accessible formats ready for analytics. This can be a combination of data processing clubbed with categorization and validation, and data transformation for improving the data quality. Data scientists, at most of the organizations, are usually busy preparing quality data for analytics, and end up with very less or no time to invest in doing actual modeling and analytics to deliver the relevant, actionable insights that companies are looking for.
It should not come to you as a surprise, as the numbers are not new, but because you faced one of the scenarios mentioned above. This might enlighten your organization as to what exactly goes into the process, and what is it that eats up so much of data analysts time. Not only this. It will also help you understand the importance of predictive data preparation in making your analytics initiative a success.
Predictive data preparation
Data preparation, in this case, is everything starting from data collection to data entry, and processing of structured and unstructured data, followed with categorization and validation to convert it into a form that is suitable for predictive analytics. Suitable here means the data is clean, complete and quantifiable. It is a cumbersome process and needs humans with data intelligence to complete it. In order to support specific analytics goals, companies must strategies their moves starting with, data collection from disparate but necessary, relevant and appropriate data sources to imaging, streamlining, thorough indexing and validation of data. Remember, data analytics is not for datasets full of ‘white noise‘.
Identifying data sources usually form a part of the data preparation process, because if the data which is being collected is not capable of delivering the result an organization is looking for, it turns out that the company is required to expand its data sources – most probably outside the enterprise. Analytics normally includes internal data sources such as transactional systems for eCommerce sites, ERP systems, data warehouses, departmental data marts, data lakes etc. Then with help from ETL, extract-transform-load; data scientists transform datasets into a form which is compatible with the needs of predictive analytics. Companies should gear up to leverage advanced analytics before it becomes the norm.
3 strategic moves data scientists make for predictive data preparation:
1. Streamlined data access
Building robust data pipeline, the first strategic move; is all about efficiently yet quickly refreshes data sets used for the predictive purpose. Reprogramming machine learning algorithms to improve accuracy becomes mandatory. If data access process if tiresome, the entire process will suffer miserably. Over a period of time, the number of data formats is going to increase and not decrease; so access to data should be robust and flexible enough to accommodate new data formats and data structures.
2. Garner data transformation expertise
As mentioned above, data size and complexity is bound to grow leaps and bounds. Data sorting, data merging, data aggregation, data reshaping, data portioning, data validation, data classification and coercing data types will have to be taken care with utmost priority. Supplementing data, adding longitude and latitude data for geospatial analysis, is something that is emerging like anything, which also needs to be accommodated. Organizations should give a serious thought wrt the expertise, whether they should hire data scientists or should take the assistance of data management solutions providers.
3. Statistical data analytics
Predictive data preparation also needs data scientists to perform exploratory data analytics to gain insights from data. Tools used for simple statistics such as calculating mean, variance, and standard deviation would be on the requirement list, followed with tools for analysis of the underlying probability distributions and variable correlations.
Statistical tools available come with trial periods, not sufficient enough to allow you to ensure that the functions meet your personal as well as business goals. So it is completely up to the company whether to go ahead with buying a costly licensed version of tools or hand over the process to data analysts with domain expertise, deep industry knowledge & scalable operations.
Contribution of data quality to business and analytics
Data and the insights in form of information derived have far-reaching effects across the enterprise; which companies know for sure. However, due to some or other reason, they get reluctant in implementing robust quality data preparation process. They at times fail to understand how critical it is that data scientists prepare and check the data accordingly to ensure that it is complete and accurate.
The C-suite decision makers of any organization though are appreciative of the objective and reliable data and insights; are most of the times unaware of the intricate details involved in predictive analytics, layers of technology and data supporting their decisions. In order to leverage data as a strategic asset, they must build confidence in the in-house data first. Quality data increases confidence, revenues, reduces costs and gives higher productivity. There is no technology that can replace data scientist’s job of cleansing and normalizing data to ensure its quality.
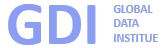